RNA Sequencing Experimental Design: Key Insights

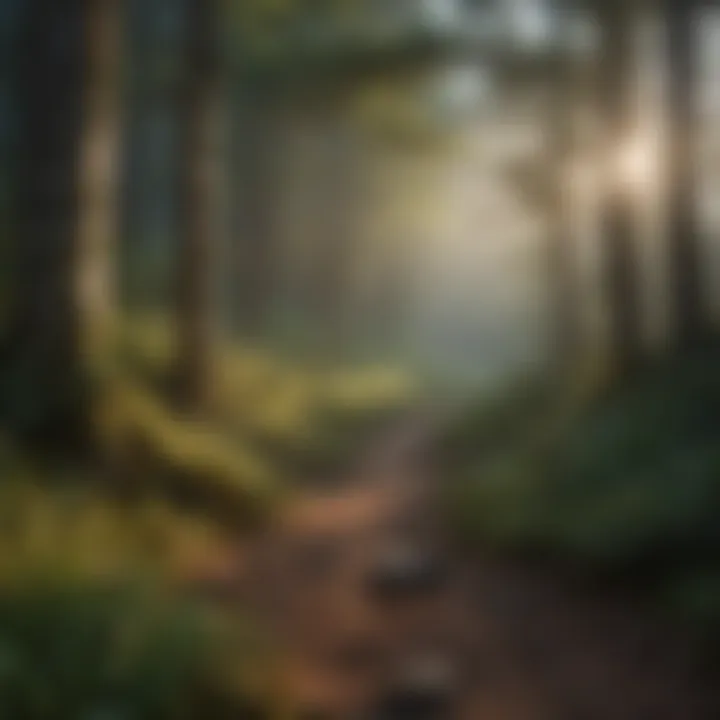
Intro
The field of RNA sequencing is advancing rapidly, providing researchers with powerful tools to explore gene expression and regulation. Designing a successful RNA sequencing experiment requires careful consideration of many factors. This article aims to dissect the essentials of RNA sequencing experimental design, highlighting key components such as sample selection, the choice of controls, and the need for replicates. By understanding these aspects, one can enhance the robustness and reproducibility of their research outcomes.
Understanding RNA Sequencing Design
Importance of Experimental Design
Experimental design in RNA sequencing is crucial for producing reliable and interpretable results. A well-structured approach helps mitigate variabilities and biases that could compromise the conclusions drawn from the data. Key areas of focus include:
- The formulation of clear hypotheses to guide the research.
- The selection of appropriate sample types and sizes.
- The inclusion of sufficient replicates to ensure statistical power.
It is essential to consider the biological variation inherent in different samples. For instance, samples from different tissues or developmental stages necessitate distinct approaches to achieve meaningful comparisons.
Sample Selection Criteria
Choosing the right samples is fundamental to the success of RNA sequencing projects. Important considerations include:
- Biological Relevance: Selecting samples that are relevant to the study question ensures that the data obtained will be applicable and insightful.
- Quality and Integrity: High-quality RNA is paramount. It must be intact and free from degradation to yield reliable sequencing results.
- Diversity within Samples: Including samples with various biological backgrounds can enhance the generalizability of findings.
Additionally, itβs beneficial to document the source and handling of samples meticulously. This practice aids in reproducibility and transparency in research.
Selection of Controls and Replicates
Incorporating appropriate controls is vital in any experimental design. Control samples help in differentiating between genuine biological variations and noise. Some strategies to consider include:
- Negative Controls: These help to detect non-specific signals in the sequencing data.
- Positive Controls: Using known samples can aid in validating the sequencing process.
- Biological Replicates: Including multiple samples from the same condition ensures that the findings are robust.
Moreover, planning for technical replicates can also be beneficial, as they provide insight into the repeatability of the measurements.
Analytical Considerations
Post-experimental analysis is as critical as the design itself. Researchers often face pitfalls when analyzing RNA sequencing data. It is essential to employ the right statistical methods to interpret results accurately. Some key points to consider are:
- Normalization Techniques: Different libraries may have biases, so effective normalization is necessary.
- Choice of Software: Leveraging reliable bioinformatics tools can streamline the analysis.
Understanding common pitfalls can help avoid misleading interpretations of data. Itβs imperative to consult current literature for guidance on best practices in RNA-seq data analysis.
"A comprehensive approach to RNA sequencing design ensures that biologically relevant data is produced, increasing the reliability and validity of the research outcomes."
Ending
Effective RNA sequencing experimental design is a cornerstone of high-quality research. By paying close attention to sample selection, controls, replicates, and analytical strategies, researchers can significantly improve the reliability of their studies. As this field continues to evolve, ongoing education and adaptation to new techniques will be key for researchers aiming for excellence in their RNA sequencing projects.
Prelude to RNA Sequencing
RNA sequencing represents a pivotal advancement in our understanding of gene expression and molecular biology. As researchers seek to unravel the complexities of RNA transcript profiles, the importance of grasping the fundamentals of RNA sequencing cannot be overstated. This section introduces the essential elements of RNA sequencing and sets the stage for a deeper exploration of experimental design considerations.
Overview of RNA Sequencing Technology
RNA sequencing, often referred to as RNA-seq, leverages high-throughput sequencing to reveal the quantity and sequences of RNA in a sample. The technology has revolutionized transcriptomics, providing insights that were previously unattainable with older methods like microarrays.
The basic workflow of RNA-seq involves several key steps:
- Sample Preparation: RNA is extracted from biological samples, which can include tissues, cells, or organisms.
- Library Construction: The extracted RNA is converted into complementary DNA (cDNA), followed by the addition of sequencing adapters.
- Sequencing: The RNA library is then sequenced on a high-throughput sequencer, generating raw data in the form of sequence reads.
- Data Analysis: After sequencing, complex bioinformatics analyses are conducted to quantify gene expression and identify differentially expressed genes.
Understanding this workflow is critical for designing RNA-seq experiments. Each step presents specific challenges and considerations that can influence the quality and reproducibility of results. Researchers must be attentive to details, such as the quality of RNA extracted and the type of library preparation kits used, as these factors directly affect data yield and accuracy.
Applications of RNA Sequencing
RNA sequencing has numerous applications across various fields. Its versatility makes it a powerful tool for exploring gene expression in many biological contexts. Some notable applications include:
- Differential Gene Expression: RNA-seq enables the comparison of gene expression levels between different conditions, such as diseased vs. healthy tissues.
- Alternative Splicing Analysis: It allows researchers to investigate the different splicing variants of genes, shedding light on their regulatory mechanisms.
- Non-coding RNA Discovery: RNA-seq can identify and quantify various non-coding RNAs like long non-coding RNAs and microRNAs, expanding our understanding of gene regulation.
- Single-Cell Transcriptomics: Recent advancements permit RNA-seq to be conducted at the single-cell level, providing insights into cellular heterogeneity within tissues.
The expansive potential of RNA sequencing is a key driving factor for its growing adoption in both academic and industry research. As scientists continue to leverage this technology, the relevance of meticulous experimental design becomes increasingly apparent. Proper design maximizes the potential of RNA-seq studies, enhancing reliability and enabling accurate biological interpretations.
"By carefully considering the steps involved in RNA sequencing, researchers can design experiments that yield meaningful and reproducible data."
In summary, this introductory section highlights the significance of RNA sequencing technology and its wide-ranging applications. Understanding these foundational elements is imperative for successfully navigating the complexities of RNA-seq experimental design.
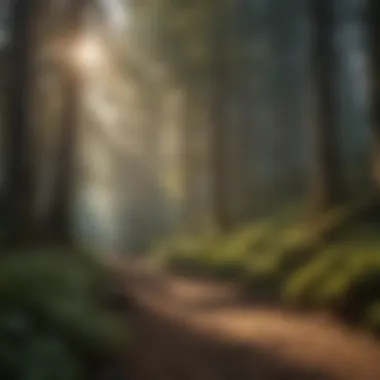
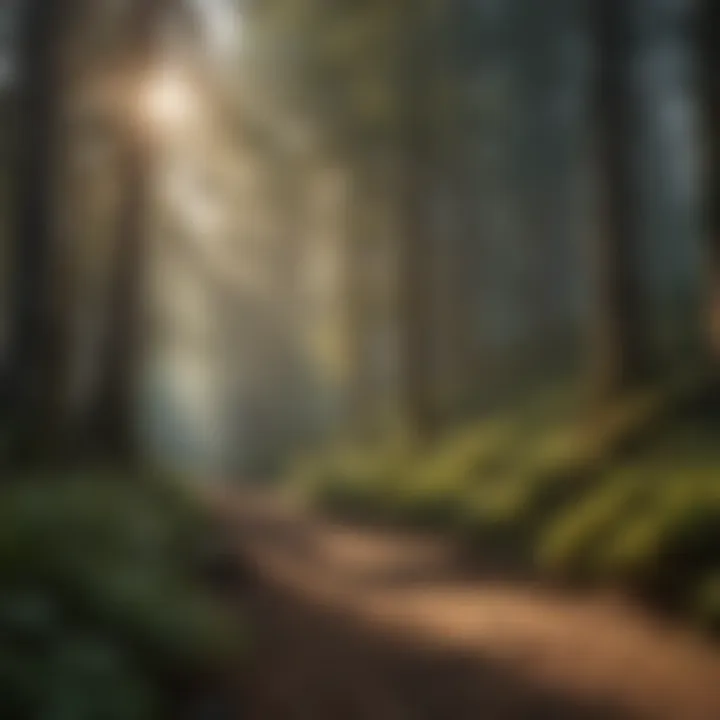
Fundamentals of Experimental Design in RNA Seq
Experimental design is a foundational aspect of any scientific study, including RNA sequencing. The integrity and reliability of research findings are largely influenced by how well the experimental design is formulated. In RNA sequencing, the complexity of biological systems demands a thoughtful and systematic approach. Without proper design, results may be skewed, leading to incorrect conclusions.
Definition and Importance of Experimental Design
The term experimental design refers to the arrangement of conditions under which experiments are conducted to gather data. It encompasses everything from the selection of samples to the methods of analysis. In RNA sequencing studies, a well-defined experimental design is crucial. It not only ensures the generation of high-quality data but also allows for valid comparisons and interpretations.
A solid experimental design reduces variability and maximizes the reproducibility of results. It also aids in identifying the biological significance of observed changes in RNA expression. By establishing a clear framework, researchers can make informed decisions about sample size, replication, and controls, ultimately yielding more reliable outcomes.
Key Components of Experimental Design
Research Question
The research question serves as the foundation of any scientific study, influencing every aspect of the design process. A well-formulated question directs the research towards specific hypotheses, ensuring that the subsequent steps are relevant and targeted. This specificity allows researchers to focus their efforts on critical areas, ultimately enhancing the precision of their study.
A focused research question facilitates the selection of appropriate experimental tools and methods, making it a beneficial and necessary choice in RNA sequencing contexts. It not only delineates the scope of the study but also informs the criteria for sample selection. Nevertheless, overly broad or vague questions can lead to confusion and dilute the relevance of the study, which presents a unique challenge.
Objectives
Objectives define the intended goals of the study, providing a roadmap for achieving the desired outcomes. Clear objectives enhance the focus of the research, ensuring that each step taken is aligned with the overall aim. They help streamline the processes involved in RNA sequencing experimental design, from data collection to analysis.
One of the key characteristics of well-defined objectives is their ability to transform broad research questions into actionable tasks. This transition enhances clarity and efficiency, making objectives a highly beneficial element of the experimental design. However, if they are not phrased correctly, they can limit the scope of discovery and innovation in research.
Hypothesis
The hypothesis is an educated guess that offers a potential explanation for the phenomena being studied. In RNA sequencing, a carefully crafted hypothesis guides researchers in the selection of methods and data analysis techniques. It is a fundamental aspect of the scientific method, influencing the investigative process and framing expected outcomes.
The hypothesis fosters critical thinking and scientific inquiry. It aligns with the objectives of the study and provides direction. However, an overly ambitious or unfounded hypothesis can lead to frustration if the experimental results do not support it. A balanced and well-considered hypothesis can therefore be an asset, but it requires careful formulation to avoid pitfalls.
Types of Experimental Designs for RNA Sequencing
Understanding the types of experimental designs for RNA sequencing is crucial for the successful generation of impactful research findings. Experimental design influences the quality, reproducibility, and interpretability of results. It is essential to select an appropriate design based on research objectives and hypotheses.
Comparative Designs
Comparative designs are fundamental in RNA sequencing studies as they allow researchers to examine the differences between groups.
Control vs. Treatment Groups
A typical setup involves contrasting control and treatment groups. The control group serves as a baseline, while the treatment group receives an intervention or condition the researcher aims to study. This design is advantageous as it provides clear insights into the effects of treatment. The key characteristic is its ability to isolate specific variables.
One unique feature of control versus treatment groups is the straightforward interpretation of results. This clarity can lead to more robust conclusions. However, one disadvantage includes the necessity for larger sample sizes to achieve statistically significant results. Without sufficient statistical power, conclusions may remain inconclusive or misleading.
Paired Samples
Paired samples represent another comparative design which measures two related samples. The main contribution of this method is its capability to control for inter-subject variability. By comparing two variables from the same subject, the design reduces noise in the data.
A significant characteristic of paired samples is their focus on individual differences. This method allows researchers to track changes more precisely. Despite its benefits, a limitation of paired samples is that it applies mainly to studies where two measurements from the same source are feasible. This situation might not always be achievable in broader RNA sequencing applications.
Longitudinal Studies
Longitudinal studies collect data from the same subjects repeatedly over time. This design is particularly valuable for understanding changes in gene expression across different time points or conditions. The data derived can add layers of complexity, allowing researchers to derive trends that may go unnoticed in a one-time snapshot.
A primary benefit of longitudinal designs is their potential for studying temporal changes, making them particularly useful in developmental biology or disease progression studies. The challenge, however, is maintaining subject consistency over time and dealing with the increased logistics and analysis complexity.
Cross-Sectional Studies
Cross-sectional studies observe a specific population at a single point in time. While they do not capture changes over time like longitudinal studies, they provide valuable insights into the relationships among variable levels of expression. This design is efficient as it requires less time and fewer resources.
One key advantage of cross-sectional designs is that they can be implemented relatively quickly, providing snapshots that can inform immediate needs or hypotheses. However, the inability to track changes can limit the depth of insights related to causality and trends.
Sample Selection and Preparation
Sample selection and preparation represent foundational elements in the design of RNA sequencing experiments. The choices made in these stages can significantly influence the outcomes, validity, and interpretability of the results. By carefully considering the criteria for sample selection and employing rigorous preparation protocols, researchers can enhance the quality of their RNA sequencing projects.
Criteria for Sample Selection
Species Considerations
Species considerations are crucial when selecting samples for RNA sequencing. The genetic and physiological characteristics inherent to specific species deeply influence gene expression profiles. This relevance makes certain species more beneficial choices, especially in studies focused on comparative genomics or evolutionary biology. For instance, using Homo sapiens allows for insights directly applicable to human health, while Mus musculus serves as an excellent model organism for many biological studies.
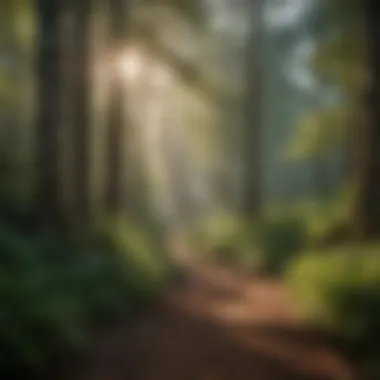
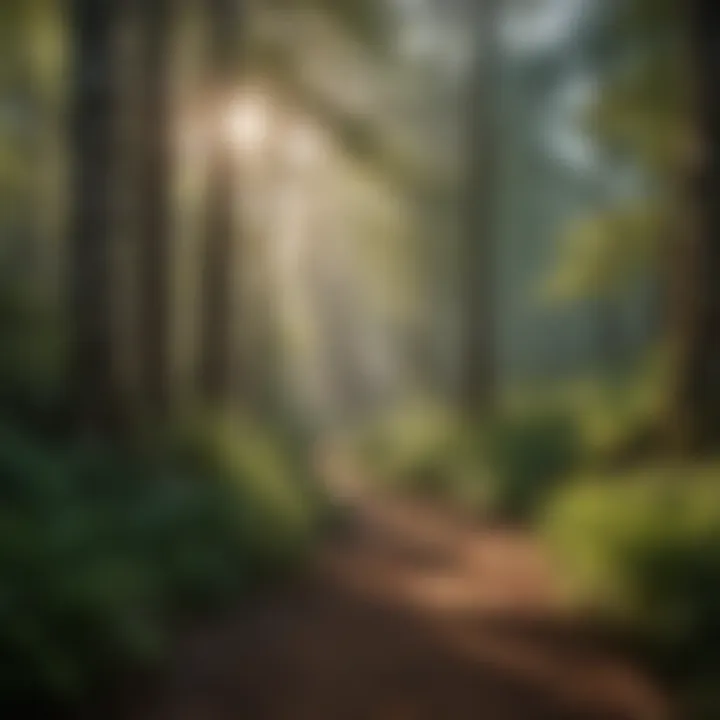
Unique features of species considerations include adaptability to laboratory conditions and availability of reference genomes. Availibility of well-annotated genomes streamline the analysis of RNA sequencing data. However, this choice has its drawbacks, as some species may not accurately reflect human disease processes.
Biological Replicates
Biological replicates are essential for ensuring that the findings of RNA sequencing experiments are robust and reproducible. These represent independent biological samples originating from different organisms but sharing the same environmental or treatment conditions. Their inclusion in experimental design helps to account for variability that may arise in biological systems.
A key characteristic of biological replicates is their ability to capture natural variation within populations. This approach enhances the generalizability of findings, making them more applicable across broader contexts, especially in biomedical research. Notably, including biological replicates helps establish confidence in the data results. However, determining the appropriate number of replicates may require careful statistical considerations and resources.
Sample Collection Methods
Sample collection methods vary according to the type of organism and the specific research objectives. Each method must prioritize the preservation of RNA integrity, as degradation can lead to misleading results. Common sample collection methods include tissue biopsies, blood draws, and harvesting cells from cultures. The choice of method can affect both the quantity and quality of the RNA obtained.
Consideration must also be given to the time of collection, storage conditions, and extraction efficiency. Implementing standardized protocols across all samples greatly assists in minimizing variability and ensuring the reproducibility of results.
RNA Extraction Techniques
RNA extraction techniques are critical in transforming the collected biological samples into usable RNA sequences. The methods employed to extract RNA should optimize yield while preserving the quality of the RNA extracted. Common techniques include using commercial kits like TRIzol or Quick-RNA Miniprep Plus. Other methods may involve mechanical disruption or enzymatic lysis to facilitate RNA release from cells.
A unique feature of these techniques is their variability in sensitivity and specificity. Some methods are better suited for specific sample types, making it essential to choose based on the nature of the sample and the intended downstream applications. Moreover, appropriate validation of extraction protocols ensures consistent results across experimental setups.
Selecting proper methods for sample collection and RNA extraction is pivotal in achieving reliable RNA sequencing data. By prioritizing quality, researchers ensure their findings contribute meaningfully to the scientific community.
Importance of Replication and Controls
In RNA sequencing studies, the concepts of replication and controls are critical. They ensure that the results achieved are both valid and reliable. Without replication, any observed results can be attributed to random variations or biases, ultimately undermining the validity of the conclusions drawn from the data. Controls, on the other hand, provide a benchmark against which experimental findings can be compared. Together, replication and controls reinforce the reproducibility of RNA sequencing results, which is essential for scientific credibility.
Biological Replicates
Biological replicates are crucial for capturing the natural variability that exists within biological systems. They consist of independent samples taken from different organisms or different biological conditions. Their primary benefit is to help identify genuine biological differences instead of variations due to stochastic processes. With biological replicates, researchers can better generalize findings across populations or conditions.
When designing experiments, it is essential to determine the appropriate number of biological replicates to use. Insufficient replicates can lead to Type I or II errors in statistical analysis. Thus, accounting for variability and ensuring an adequate sample size can significantly contribute to the robustness of conclusions drawn from RNA sequencing studies.
Technical Replicates
Technical replicates involve repeated measurements of the same biological sample. They serve to assess the precision of the experimental techniques employed. The key characteristic of technical replicates is that they account for variability introduced by the measuring process itself. This includes aspects like sample handling and sequencing accuracy. By including technical replicates, researchers can identify anomalies that may arise from the sequencing process and correct for these discrepancies in data interpretation.
While technical replicates increase confidence in the data, it is important not to confuse them with biological replicates. The role of technical replicates is to provide thorough and precise data on a sample, rather than capture biological variability.
Control Samples
Control samples play an essential role in RNA sequencing experiments. They are used as references to compare the experimental samples against and help in identifying any confounding influences that may affect the results.
Negative Controls
Negative controls are samples where the expected outcome is not present, serving as a baseline for comparison. One significant aspect of negative controls is their ability to highlight potential false positives. If a signal is detected in a negative control sample, it may indicate contamination or other artefacts in the experimental setup.
The key characteristic of negative controls lies in their ability to establish a clear standard for what represents a 'background' signal. This makes them a beneficial choice in many RNA sequencing studies. Their unique feature, being devoid of the target of interest, allows researchers to discern between true signals and any noise generated during experimentation. However, care should be taken in interpreting the absence of signals, as it may not always mean a valid negative result.
Positive Controls
Positive controls contain all the necessary components to ensure the expected response is observable. This establishes that the experimental conditions and methodologies employed are functioning properly. A major benefit of positive controls is they can help assess the efficiency and accuracy of the entire experimental setup.
The key characteristic of positive controls is that they are expected to yield a specific response, which can help validate the assay's integrity. They serve as a benchmark to which experimental results can be compared. While beneficial, over-reliance on positive controls can sometimes lead to a false sense of assurance in results if not appropriately placed within the context of biological relevance.
"Replication and controls are the bedrock of trustworthy RNA sequencing results."
Ultimately, the combination of biological and technical replicates, alongside carefully chosen control samples, creates a robust experimental design that enhances the credibility and reproducibility of RNA sequencing studies.
Data Analysis Considerations
Data analysis is a crucial stage in RNA sequencing experimental design. It involves processing the complex results generated from sequencing experiments to derive meaningful biological insights. The success of RNA-seq studies greatly hinges on how well data is analyzed. Proper data analysis ensures that the findings are both robust and reproducible, which is essential for scientific credibility.
Overview of RNA-seq Data Analysis
RNA-seq data analysis typically encompasses several steps, beginning with raw data quality assessment, trimming of sequence reads, and then aligning those reads to a reference genome or transcriptome. Following alignment, various computational tools are used to quantify gene expression levels. These workflows are essential to identify differentially expressed genes. The entire process analysis must be well planned and executed to provide viable results that can lead to further biological discoveries.
Normalization Techniques
Normalization techniques are pivotal in RNA-seq data analysis as they aim to correct systematic biases that can arise during the sequencing process. These techniques account for variations in sequencing depth across different samples. Common normalization methods include TMM (Trimmed Mean of M-values), RPKM (Reads Per Kilobase of transcript per Million mapped reads), and TPM (Transcripts Per Million). Each of these methods helps to ensure that gene expression levels can be accurately compared across different samples, yielding reliable insights into biological processes. Without proper normalization, the results could be misleading, as differences in raw data may not accurately reflect true biological variations.
Statistical Analysis Methods

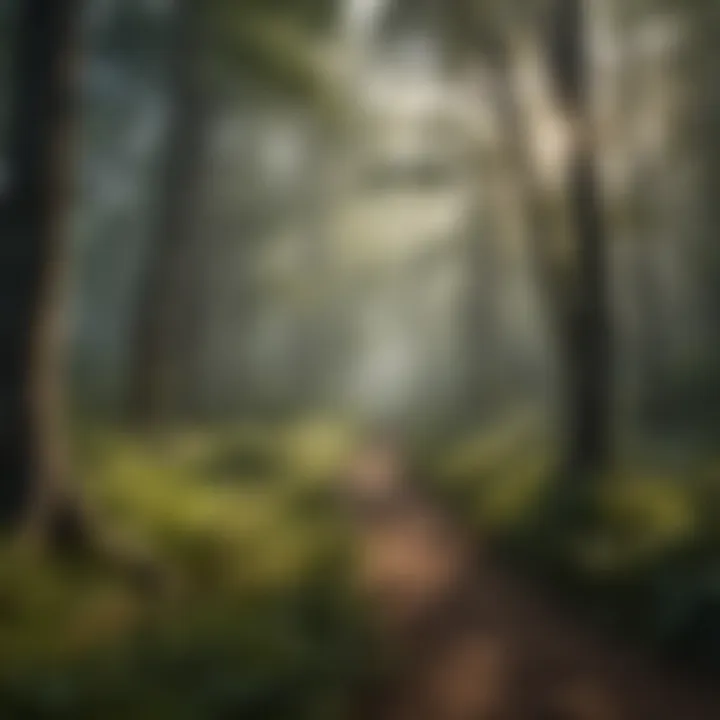
Statistical analysis methods play a fundamental role in interpreting RNA-seq data. There are various approaches for analyzing the data, among which two main methods are noteworthy: differential expression analysis and pathway analysis.
Differential Expression Analysis
Differential expression analysis focuses on identifying genes whose expression levels differ significantly between two or more conditions. This analysis allows researchers to pinpoint biological changes that may be relevant to experimental conditions. A key characteristic of differential expression analysis is its statistical rigor and ability to handle large datasets. It is a beneficial approach because it can highlight not just significant changes, but also quantify the extent of those changes across different conditions. Its unique feature lies in its ability to provide a list of genes ranked by expression level changes, which is essential for downstream interpretations. While powerful, it also has its limitations, such as the potential to produce false positives if not properly controlled with multiple testing corrections.
Pathway Analysis
Pathway analysis is another critical component of RNA-seq data analysis. This approach focuses on evaluating how differentially expressed genes are involved in specific biological pathways. It links gene expression data to biological functions and can provide deeper insights into the underlying mechanisms driving observed changes. A key feature of pathway analysis is its capacity to summarize complex data into actionable biological insights. This makes it a popular choice among researchers who seek to understand the implications of their findings in a broader biological context. Nonetheless, it comes with challenges, such as the dependency on existing biological knowledge and databases, which may not always cover all relevant pathways.
Understanding data analysis considerations enhances the robustness of RNA sequencing studies and can significantly influence the outcome of subsequent research.
Common Pitfalls in RNA Sequencing Experimental Design
In the realm of RNA sequencing, experimental design is pivotal. Even minor mistakes can lead to significant issues. Understanding the common pitfalls can enhance the overall quality of research. This section emphasizes these pitfalls and their consequences, providing insights to help researchers navigate them more effectively.
Inadequate Sample Size
One of the most frequent errors in RNA sequencing experiments is having an inadequate sample size. A small sample size can greatly reduce the statistical power of your results. If the sample is not representative of the larger population, generalization of findings may be misleading.
- Statistical Power: With fewer samples, it's harder to detect true biological differences. This can lead to missing important findings.
- Variability Estimates: Small samples may not capture the variability present in the larger population. For accurate representation, it is crucial to calculate adequate sample sizes prior to the experiment.
Consider using power analysis tools to determine the minimum sample size needed to yield reliable results. This exercise is essential in ensuring that your conclusions are robust and scientifically valid.
Neglecting Controls
Control samples are essential in RNA sequencing experiments. Neglecting to include appropriate controls can compromise the integrity of the study's findings. Controls allow researchers to differentiate between specific effects and background noise in the data.
- Types of Controls: Negative controls help identify background noise, while positive controls ensure the detection system is functioning correctly. If these are overlooked, interpreting results can become a challenge.
- Comparative Analysis: Without controls, determining the significance of observed changes becomes difficult. Ensure to include both types of controls in your experimental setup to validate your findings and improve reliability.
"A well-designed control can make a significant difference in the interpretation of RNA sequencing results."
Improper Data Interpretation
Data interpretation is often a decisive final step in RNA sequencing studies. Misinterpretation can arise from various factors, such as incorrect statistical methods or overlooking data normalization processes.
- Statistical Methods: It's vital to select appropriate statistical analyses pertinent to the datasets. Incorrect methods can lead to skewed conclusions.
- Normalization Procedures: RNA sequencing data requires normalization to adjust for biases introduced during library preparation and sequencing. Ignoring these adjustments can yield misleading results and affect downstream analyses.
Careful scrutiny and validation of the interpretation process are paramount. Engaging in peer discussions or consultations can provide further clarity in interpreting complex data sets.
Future Directions in RNA Sequencing Experimental Design
The field of RNA sequencing continues to evolve, with novel techniques and methodologies emerging that propel research forward. Understanding the future directions in RNA sequencing experimental design is crucial for researchers aiming to optimally leverage these advancements. The significance of exploring upcoming innovations lies in the ability to enhance data accuracy, reduce costs, and expand the applications of RNA sequencing in diverse fields, including plant and forestry sciences. With the increasing push for reproducibility and robustness in experimental results, aligning research design with these future trends can significantly benefit study outcomes.
Innovative Techniques in RNA Sequencing
As technology improves, various innovative techniques in RNA sequencing are gaining traction. These methodologies focus on increasing sensitivity, specificity, and throughput. Some notable advancements include:
- Single-Cell RNA Sequencing (scRNA-seq): This technique allows for the examination of gene expression at the individual cell level. Understanding cellular heterogeneity enhances insights into complex biological systems, which is particularly relevant in studies of forest ecosystems where individual cell responses can vary widely.
- Spatial Transcriptomics: This approach combines histological analysis with RNA sequencing to maintain the spatial context of gene expression in tissues. Incorporating this method into experimental design aids in understanding the spatial organization of gene activity across forest species, contributing to habitat and ecological research.
- Long-Read Sequencing Technologies: Technologies such as PacBio and Oxford Nanopore provide long-read capacities that improve the ability to resolve complex genomic regions. They can enhance the discovery of isoforms and structurally variant transcripts, enriching the data set available for analysis.
These innovative techniques open new avenues for research, enabling deeper insights into gene expression and regulation across different species and ecosystems.
Integrating Multi-Omics Approaches
The integration of multi-omics approaches is becoming increasingly essential in RNA sequencing experimental design. By combining RNA sequencing with other omics technologies, such as proteomics and metabolomics, researchers can gain a comprehensive understanding of biological processes. This integration permits elucidation of the interplay between genes, proteins, and metabolites, facilitating a multi-dimensional view of cellular functions.
Benefits of integrating multi-omics approaches include:
- Holistic Insights: By examining the relationships between RNA, proteins, and metabolites, researchers can better comprehend cellular responses to environmental stressors, which is crucial for forestry professionals studying how trees respond to climate change.
- Improved Data Validation: Multi-omics data can validate findings across different layers of biological information, increasing credibility.
- Enhanced Resolution in Data Analysis: Combining datasets helps address limitations seen when analyzing single omics data alone. Statistical models can become more robust, providing clearer pathways for understanding complex biological questions.
Integrating these methods into RNA sequencing experimental design encourages a more dynamic approach to research, allowing scientists to address intricate questions regarding biological mechanisms in forestry and other fields.
Finale
In concluding this discussion on RNA sequencing experimental design, it is essential to underscore the profound impact that meticulous planning can have on research outcomes. Experimental design in RNA sequencing is not merely an academic exercise; it is a foundational aspect that influences the validity and reproducibility of scientific investigations. A well-structured design minimizes biases, enables precise data interpretation, and ultimately enhances the quality of findings derived from RNA sequencing studies.
Summarizing Key Points
To recap, several critical elements were highlighted throughout the article:
- Defining the Research Question: Clarity in the research question guides all subsequent steps in the experimental design.
- Sample Selection and Preparation: Appropriate criteria for selecting biological samples are vital for obtaining representative data.
- Importance of Replicates and Controls: Both biological and technical replicates add robustness to the experiment, while control samples play a crucial role in validating results.
- Data Analysis Techniques: Understanding the nuances of data analysis is critical for drawing meaningful conclusions from RNA sequencing data.
- Identifying Common Pitfalls: Awareness of common mistakes, such as inadequate sample size, can prevent significant errors in interpretation.
This summary serves as a valuable reference for researchers aiming to implement RNA sequencing effectively in their studies.
Final Thoughts on RNA Sequencing Design
As RNA sequencing technology continues to evolve, so too must our approach to experimental design. Incorporating innovative techniques and multi-omics strategies can enhance our understanding of complex biological systems. Researchers are encouraged to remain adaptable and embrace new methodologies while adhering to the fundamental principles of sound experimental design. Balancing rigor with creativity will foster advances in RNA sequencing that are both impactful and reproducible. The road ahead holds great promise for those who approach RNA sequencing with diligence and an open mind.